Statistics for Beginners
Usually statistic is a difficult matter, specially for biologist. To ask mathematicians resolves not always the problem as the mathematicians not always understand what the bioloists wants to say... and vice versa. Another problem, specially with modern computer programs, is that you can easily apply different statistics on your data, but these programs usually don't warn you, if this statistical method is allowed. So here are some hints about statistics, many ideas I got from Steve's Place, but unfortunately this site is now down. Here now I am citing, what I have copied from him:
Numbers
Results and statistics should show your data in whatever format is most appropriate. Tables are dull, but sometimes necessary: if you can beat a figure, diagram, bar chart, pie chart, histogram, cladogram, photo, or anything else graphic out of your data, so much the better.
When quoting numbers, don't quote them to a squadrillion decimal places that you couldn't possibly justify. In general, you're unlikely to be working to more than 3 significant figures, and probably less, so recognise this and write your numbers accordingly. I never want to see 4.0245635324 mm as the average length of a maggot, when you have only measured them to the nearest millimetre. this means,never write the measured temperature was 30.55 °C when your sensor has a precision of only 0.1 °C.
Statistics
Statistics are always fraught. Although you are always encouraged to do statistics on data, I have been in a situation where I was asked to perform stats on a sample size of one or two, where the data was complete dross, and the method obviously flawed.
Sample size
That said, if you can do stats, do them. Depending on how many factors you are investigating, you will need various sample sizes. To get a reliable mean value for an ecological sample, a sample size of less than 30 is not recommended, to compare two biochemical assays, five samples from each assay under well controlled conditions is probably OK. In general, ten data points per 'line' on a line graph or scatterplot, or five per bar on a bar chart is reasonable. This is something I wish more lecturers in universities would do, as I was constantly annoyed by those who would have us study three soil types combined with four wood preservatives, combined with two wood species, combined with six species of fungus, but didn't notice (or care) that this left you with only enough time to replicate each of these 144 combinations once, making stats extremely unreliable, and the experiment a disappointing waste of time.
Which statistic?
On a similar topic, chosing the correct statistics to analyse your data is not the simple matter you might think. The bog-standard techniques are taking means, using the the t-test or Χ2-test and performing linear regression. The advanced techniques are things like the Mann-Whitney U-test, analysis of variance, analysis of covariance, analysis of deviance and nonlinear regression. These are not interchangeable, and I have seen t-tests used to analyse some quite utterly inappropriate things. The important thing to understand is the underlying error structure of your data.
Normally distributed data fits the nice bell shaped Gaussian curve you've all seen before. Normally distributed data is unbounded (i.e. can go from -8 to +8), symmetrical, and various other things. To take the mean and standard deviation of data, or to perform a t-test, or an analysis of variance, is to assume a normal error structure for your data. If your data is not normally distributed (or not near-as-damn-it normally distributed), you should not use these statistics. In particular, if such (faulty) analysis is only borderline significant, and you know that the error structure is non-normal, then you should not have much faith in your analysis.
Poisson distributed data is count data. It is bounded (i.e. can only go from 0 to +8), not symmetrical about its mean, and should not be analysed with t-tests. If you have counted the number of nematodes in a turd, or the number of people kicked to death by horses per year, you have Poisson distributed data. You can use analysis of deviance to analyse such data (if you specify the error structure), but only with the posher sort of stats analysis package. Alternatively, to analyse count data in various categories, you can use the Χ2-test, which is useful to see if (for example) the observed ratios of phenotypes in a simple genetic cross match what you expect from Mendelian genetics. However, don't use this test if any of the categories have fewer than four tallies in them, or the test will go funny.
Binomially distributed data is percentage data. It is strictly bounded (i.e. it can only go from 0 to 100%, or from 0 to 6, or similar), and is a pig to analyse. You need either to use analysis of deviance with a suitable error structure, or transform the data in some way so as to torture the transformed data into being normal (such as using logits or probits). Avoid if possible: unfortunately, some rather common things have this error structure, like percentage mortalities.
Sometimes you will have no idea what the error structure is: it could even have several modes, and hence, even taking a mean is worthless. In such cases you want a non-parametric test, like the U-test that relies on the median of the data, not the mean.
Regression analysis suffers from similar problems: you can only do linear regression on data that appears to fit a straight line. If it doesn't, you will need to venture into non-linear regression, and get up close and personal with techniques for making non-linear data linear (such as using logarithmic transformation of the data, or Lineweaver-Burk reciprocal plots), or using non-linear algorithms like Levenberg-Marquardt (or at least something like SigmaPlot, which does it for you).
In Nature's Instructions for Authors it is stated, that the "statistical testing should state the name of the statistical test, the n for each statistical analysis, the comparisons of interest, a justification for the use of that test (including, e.g., a discussion of the normality of the data when the test is appropriate only for normal data), the alpha level for all tests, whether the tests were one-tailed or two-tailed, and the actual P value for each test (not merely "significant" or "P < 0.5"). It should be clear what statistical test was used to generate every P value. These details should be reported briefly at the most appropriate place in the text: either in the text of a Methods section, or as part of a Table or Figure caption.
Typical errors are:
Multiple comparisons: When making multiple statistical comparisons on a single data set, it should be explained how the alpha level was adjusted to avoid an inflated Type I error rate, or appropriate statistical tests for multiple groups (such as ANOVA rather than a series of t-tests) should be selected.
Normal distribution: Many statistical tests require that the data be approximately normally distributed; when using these tests, it should be explained how was the data for normality tested. If the data do not meet the assumptions of the test, then a non-parametric alternative should be used instead.
Small sample size: When the sample size is small (less than about 10), tests appropriate to small samples should be used or the use of large-sample tests should be justified.
The really easy statistics site wrote J. Deacon from the University of Edinburgh.
Overlapping error bars:
https://www.graphpad.com/support/faq/spanwhat-you-can-conclude-when-two-error-bars-overlap-or-dontspan/
https://www.nature.com/articles/nmeth.2659
SD from 2 values:
https://www.graphpad.com/support/faqid/591/
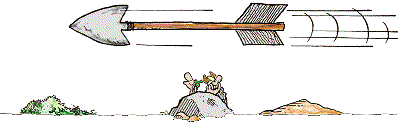
Last modified: 04.04.2010